企業如何使用內部數據建立生成式人工智能(Generative AI)? 來自Bloomberg, Morgan Stanley 和 Google 的案例
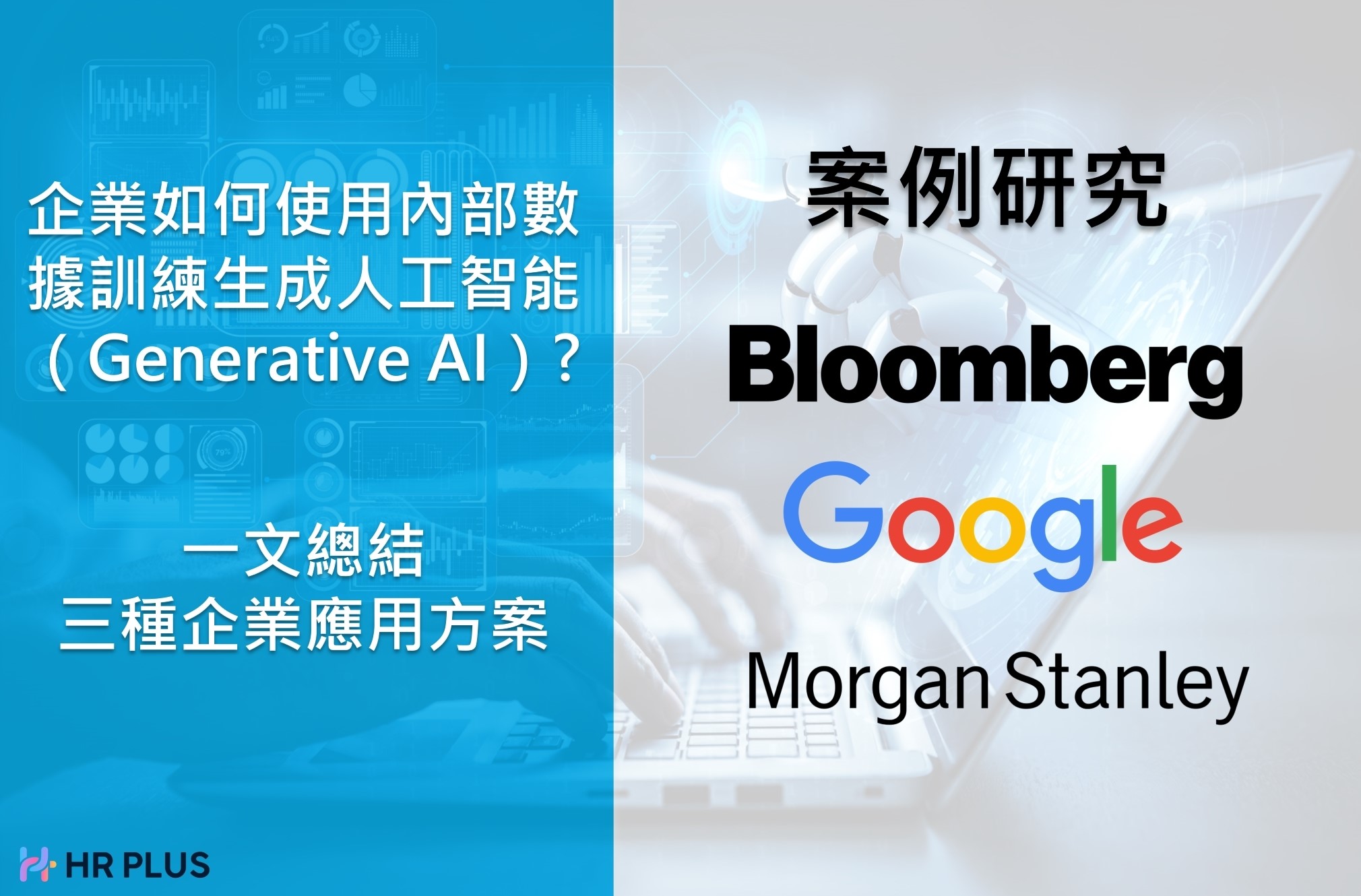
越來越多企業嘗試運用ChatGPT和其他大型語言模型 (LLM)進行實驗,以生成式人工智能優秀的表達和推理能力,將內部數據轉化為內部工具或客戶產品。這些應用包括客戶服務支援教育,為銷售人員處理產品/服務推薦,或在員工離職前保存其知識。以下我們將通過Bloomberg、Morgan Stanley和Google的三個案例研究,總結出企業在採用生成式人工智的三種常見方案。
- Bloomberg : 從零開始訓練金融類ChatGPT:
首先聲明,從零開始創建特定領域的模型並不是一種常見的方案,因為這需要大量高精度數據、強大而昂貴的算力和經驗豐富的數據科學家。以Bloomberg為例,他們在開發BloombergGPT時,Bloomberg的數據科學家結合超過40年的金融數據與大量的金融文件和互聯網文本,歸納出700億個分詞(Token)和500億個參數,過程中共消耗130萬個小時圖形處理器運算時間,才能初步創建一個針對金融領域的特定LLM模型。儘管Bloomberg未有公怖他們在項目中投入多少資金,但以Open AI 在開發Chatgpt 的成本為例,業內人士估計開發成本為最少5億美金,這還沒有計入每日70萬美金的運作成本。
- Google : 對現有LLM進行微調以訓練醫學ChatGPT:
另一種方法是將特定領域的文本添加到預訓練模型中,對現有的LLM進行微調訓練。這種方法相對於從零開始訓練所需的數據和計算時間較少。Google在開發其Med-PaLM2模型中便應用了這種方法。他們首先使用了一般的PaLM2 LLM,並結合31位醫學專家的論文作為基礎重新訓練,從而能夠回答85%的美國醫學許可考試問題。儘管有所成果,但該模型在一些基礎問題上依然有機會犯下「致命」錯誤,所以現階段不適用於臨床實踐。微調訓練有其局限性,但對於許多公司來說,這種方法的可行性相比從零開始更經濟,但仍需要投入大量資源在購買雲算力和獲取專業數據。
- Morgan Stanley : 透過提示工程(Prompt Engineering)調整現有LLM:
最後一種方法最常用,成本亦較低,即是通過提示工程對現有的LLM進行自定義調整。Morgan Stanley 的數據科學家精心挑選的10萬份文檔對GPT-4模型進行了提示調整,這些文檔包含重要的投資、一般商業和投資流程知識。 Morgan Stanley 在公司的私有雲中創建了一個僅供其員工訪問的ChatGPT工具,使得金融顧問能夠獲取準確且易於獲取的公司內部信息。這種方法的門檻不高,並且不需要大量數據,運作成本上亦有不少優勢,以另一間投資公司Moringstar 為例,他們仿傚Morgan Stanley 把 1萬份文檔對GPT-4模型進行調整,並向其金融顧問及客戶提供使用權,在使用的頭一個月裡,Mo 回答了 25,000 個問題,每個問題的平均成本約為 0.002 美元,總運作成本僅為 3,000 美元 (HKD 23,400)。
質大於量至關重要
在建立生成式AI上,除了算力成本和海量數據,高質數據和品質監督對LLM是否有效亦至關重要。以Morgan Stanley 為例,他們在菲律賓招聘了約 20 名知識管理人員,根據多個標準對文檔進行評估,以決定其是否適合集成到 GPT-4 系統中。此外,為處理生成式AI模型時有發生的會產生幻覺問題(即產生不正確或不存在的信息),Morgan Stanley 設定了400個有正確答案的“黃金問題”,並組織300名金融顧問為先鋒隊進行持續試驗,每當系統進行任何更改時,員工都會用黃金問題對其進行測試。這種嚴格的評估過程有助於保持系統回答的可靠性和準確性。
生成式AI在企業應用上的前路
儘管不少公司對生成式AI,在員工生產力和客戶體驗提高的長遠願景感到樂觀。但在現有技術上,無論採用哪種方案,將內部數據轉化為生成式AI都會面巨大的資本投入和入門門檻。業界相信隨著更多AI科技公司入場競爭,在未來3年LLM 的訓練方法和成本會有所下降,並在企業間有所普及。
____________________________________________________________________________________________________________________________________________
How can businesses leverage internal data to build Generative AI?
Insights from Bloomberg, Morgan Stanley, and Google
Companies are increasingly using generative AI tools like ChatGPT to transform internal data. These tools help capture and provide access to intellectual capital, empowering customer-facing employees with knowledge on company policies and product recommendations. They also assist in resolving customer service problems and preserving employee knowledge. Learn from case studies at Bloomberg, Morgan Stanley, and Google, and discover three common approaches to adopting generative AI in Corporate use cases.
- Bloomberg: Training a Finance-Focused ChatGPT from Scratch:
Creating and training a domain-specific model from scratch is a less common approach due to the need for a substantial amount of high-quality data, significant computing power, and skilled data science talent. Bloomberg stands as an example of this approach, where they developed BloombergGPT by combining over 40 years of financial data with extensive financial documents and internet text.
This involved dataset over 70 billion tokens and 50 billion parameters, consuming a staggering 1.3 million hours of GPU processing time. While Bloomberg has not disclosed the exact investment in this project, considering the estimated cost of at least $500 million for OpenAI's development of ChatGPT, coupled with a daily operating cost of $700,000, it underscores a significant financial commitment.
- Google: Fine-Tuning an Existing LLM to Train a Medical-Focused ChatGPT:
An alternative approach involves fine-tuning an existing LLM by adding specific domain content to a pre-trained model. This approach requires less data and computing time compared to training from scratch. Google's use of fine-tuning with their Med-PaLM2 model with the articles of 31 medical experts, resulting in improved performance on answering 85% of medical licensing exam questions. Google started with their general PaLM2 LLM and retrained it on carefully curated medical knowledge from various public datasets.
While the model demonstrated promising results, it still carries the potential for critical errors in fundamental questions, rendering it unsuitable for clinical practice at this stage. Fine-tuning has inherent limitations; nevertheless, it provides a more cost-effective solution for many companies, albeit requiring substantial resources for cloud computing and obtaining specialized data.
- Morgan Stanley: Customizing an Existing LLM through Prompt Engineering:
The most prevalent and cost-effective approach involves customizing an existing LLM through prompt engineering. Morgan Stanley's data scientists meticulously selected 100,000 documents and performed prompt engineering on the GPT-4 model, incorporating crucial knowledge related to investments, general business practices, and investment processes. Within their private cloud, Morgan Stanley created a ChatGPT tool exclusively accessible to their employees, enabling financial advisors to retrieve accurate and readily available internal information.
This approach offers a relatively low entry barrier and does not necessitate a substantial amount of data. Additionally, it provides cost advantage in operation. For example, Morningstar, another renowned investment firm, followed a similar approach by adjusting 10,000 documents for the GPT-4 model and granting access to financial advisors and clients. In the initial month of use, the system, referred as "Mo" answered 25,000 questions at an average cost of approximately $0.002 per question, resulting in a total operational cost of merely $3,000 monthly.
Quality over Quantity
When it comes to generative AI, ensuring high-quality content is crucial before customizing LLMs, much like traditional knowledge management where documents were loaded into discussion databases. Morgan Stanley provides an example of this practice, employing a group of around 20 knowledge managers in the Philippines who continuously evaluate documents based on multiple criteria to determine their suitability for integration into the GPT-4 system. Many companies without well-curated content will face challenges in achieving this level of quality for their specific purposes.
Furthermore, it is widely acknowledged that Generative AI models can occasionally produce incorrect or nonexistent information, leading to what is commonly known as "hallucinations." To ensure accurate responses, Morgan Stanley has developed a set of 400 "golden questions" with known correct answers. The system is piloted for several months by 300 financial advisors, and whenever any changes are made, employees test it against the golden questions to identify any instances of "regression" or less accurate answers. This rigorous evaluation process helps maintain the reliability and precision of the system's responses.
The Path to Leveraging Generative AI in Business
While many companies are optimistic about the long-term vision of improving employee productivity and customer experiences through Generative AI, it is important to note that the transformation of internal data into Generative AI entails significant capital investment and other entry barriers. However, the industry believes that as more AI tech companies enter the competition, the training methods and costs of LLMs will decrease in the next three years, making them more accessible to businesses.